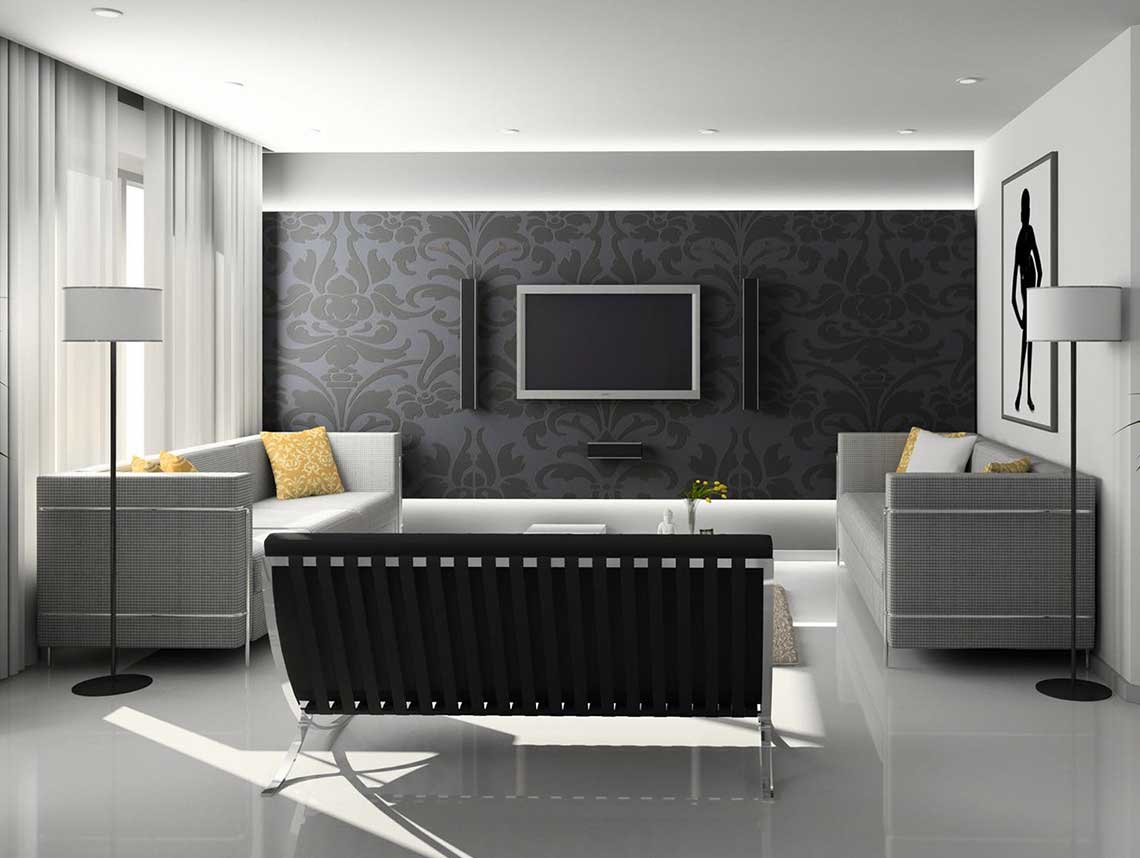
Impact of AI and ML on video streaming devices for the better TV experience
Background
Artificial intelligence (AI), with machine learning (ML) and deep learning (DL) technologies, promises to transform every aspect of businesses, devices, and their usage. In some cases, it enhances the current process, and in some cases replaces them.
As video streaming devices (including TV & STBs ) vie to be one of the AI assistants in the home. However, there are several touchpoints in regular streaming devices and Video processing that are ripe for TV transformation with AI advancements.
The quality of experience expected to increase with enhanced models visually and respect to ease of use. The video streaming devices with these features will surely outperform the ones without.
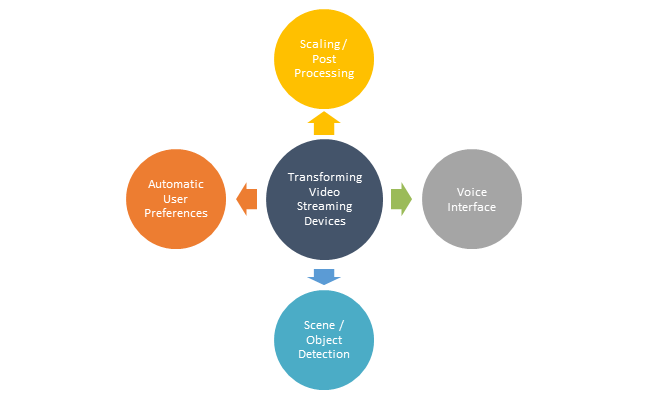
This blog discusses the use of different AI/ML models performed in video streaming devices to enhance the TV user experience.
Super Resolution
Super-resolution is a technique that enhances the quality of a given low- resolution to high resolution by upscaling. Whereas people incline too high quality and visually appealing images and video quality.
Therefore, today the super-resolution feature can be found in many video streaming display devices, producing resolutions that are 2-4 times higher than the pixel count of the sensor.
Neural Network-based scaling is performing a lot better than the traditional bilinear scaling as can be seen from the example pictures below.
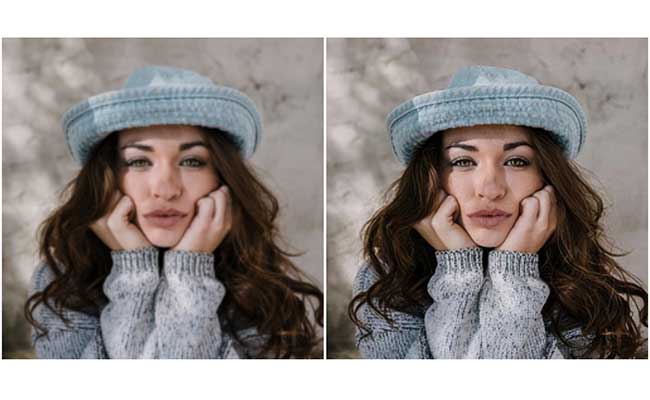
As can be seen in the pictures scaled with NN models, the details are preserved or enhanced.
Within the Neural Networks using for scaling there are two types of models –
• Convolution/Deconvolution (Deconv) based
• Generative Adversarial Network (GAN) based.
Deconv based models are simpler and generalize better, whereas the GAN based ones seem to perform very well for scaling function. The Deconv model has an initial section that is similar to familiar networks such as ResNet, which reduces the size of the image but increases the number of features and the latter part is an expansion network, which uses deconvolution operation to increase the resolution using the features. The residual connections in the network seem to help to generalize better.
Generative Adversarial Networks (GAN) consist of a system of two neural networks — the Generator and the Discriminator — dueling each other. Given a set of target samples, the Generator tries to produce samples that can fool the Discriminator into believing they are real. The Discriminator tries to resolve real (target) samples from fake (generated) samples.
Using this iterative training approach, we eventually end up with a Generator that is really good at generating samples similar to the target samples.
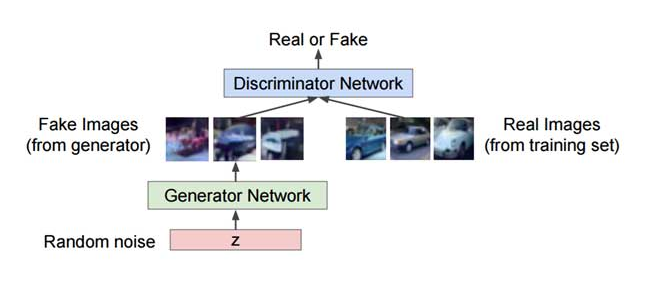
InPainting with Neural Networks
There are several cases where a part of the image is damaged or distorted or obscured with a previous watermark. Neural Network can repair such blemishes quite well by guessing the parts of the image missing. Similar to Super Resolution, Deconv networks and GANs can be accomplished.
However, Deconv networks seem to be performing better owing to the better generalization. The single network trained to do both Super Resolution and Inpainting (and other noise reduction) together.
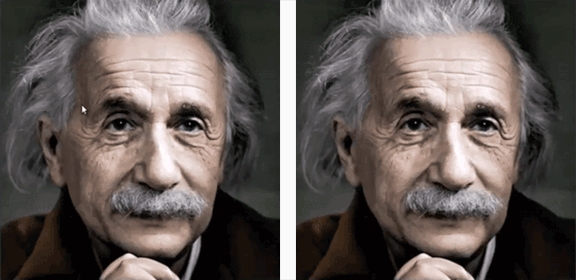
Frame Rate Conversion
Interpolation and upscaling for animated graphics is hard as it has sharper edges and simple interpolation tends to make the images blurred. These softer edges are not visually pleasing in high resolution. Again, Neural networks seem to be out-performing traditional methods in frame rate conversion (and up-sampling). The paper linked below shows how using Variational Autoencoder, GANs, and Deep Convolution Networks can be used to perform this function and Deep Convolution Networks seem to have performed better than other and traditional upsampling.
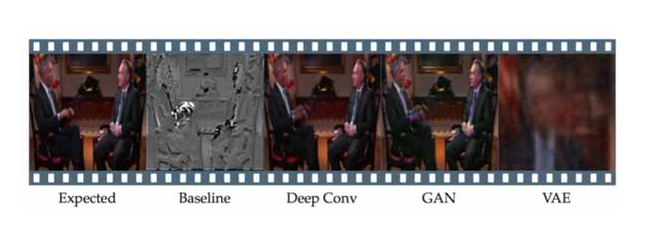
Integrated Home Assistant in TV
AI integrated Video streaming devices at home tv can know the family members watching and can keep track of each individual’s preferences, viewing habits and specific programming.
With the several applications providing content, it takes quite some time to tune to the specific programming to switch the applications and to browse through the menus.
As per the latest Nielsen report, the average viewer takes 7 minutes to pick what to watch. Just one-third of them report browsing the menu of streaming services to find content to watch, with 21% saying they simply give up watching if they are not able to make up their minds.
A background application can perform this task knowing the specific user. Video viewing through TV-connected devices has increased by 8 minutes daily, in large part due to the penetration of those devices and the falling away of older, less nimble setups.
Seven in 10 homes now have an SVOD service and 72% use video streaming-capable TV devices. Also, the report asserts that customers are not in a comfortable groove when it comes to choosing what to watch.
A background application running on TV can perform the task of recommending the specific content as per individuals’ preferences and viewing habits.
Anonymized Person Detection
Above all functions performed without intruding into the privacy of the users and without recording specific user’s video/face etc.
Also, several new AI techniques are available to anonymize incoming video streaming at the source and perform person recognition at the ball/stick level. Moreover, the NN identifies the user based on the gait of the person without intruding into privacy.
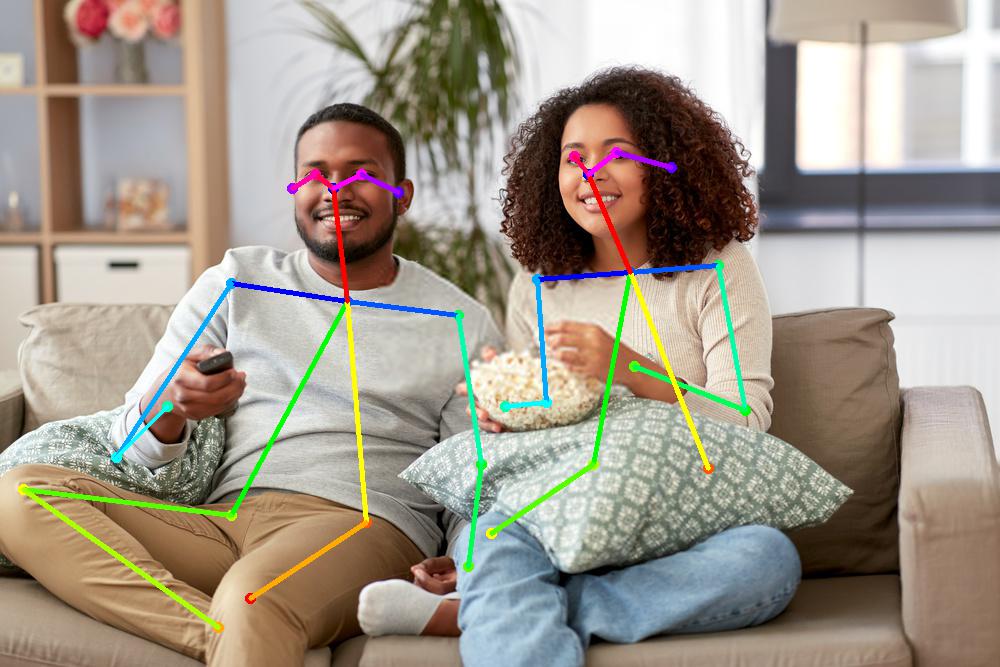
Noise Reduction
Post-processing can remove noise and improve PSNR caused by Encoders’ blocking, Blurring, Ringing and Perceptual Quantizer (PQ).
Encoder introduced impairments are removed with specific filters like deblocking filter etc. However, using Neural Networks can improve the performance of noise reduction and they all can be performed by one algorithm.
Post-processing options in TVs to perform MEMC (Most Estimation and Motion Compensation). Also, which has staunch critics, can be enhanced with Neural Networks.

Automatic Scene Detection
There are several applications that can use the information about the running image/scene. Video analytics to detect specific actions can be used to rate the specific section of the video. Similarly, voice can be analyzed for Language rating (for kid proofing).
Every frame can be classified for detection of Specific Objects/location, Face recognition of the character and Auto Classification of Nudity
The insights can be used for auto-captions for regulatory warnings such as Smoking/Drinking.
Voice Controls
Very similar to the Home AI assistants such as Amazon Alexa and Google Home, Video streaming devices at home expected to respond to voice commands. Today many remote controllers have already voice-activated.
For a high sound output device to be an input device is quite complex, having to cancel the output audio.
In addition, there will be a variable delay in the ambient noise, if the voice enhanced with external speakers. Wake-word detection and Automatic Speech Recognition (ASR) implemented using complex Neural networks today. And for TVs, the ambient noise needs to be compensated to hear the commands.
Conclusion
With proper Neural Network acceleration hardware in the TV/STBs, all or some of the above functions can be implemented for better user experience compared to the current post-processing.
In addition, this processing can alleviate the chip designers to do away with the current expensive filters and post-processing hardware for a unified hardware element to perform Neural Network processing.
One of the problems of the AI algorithm development is dataset availability and annotation. Also, for most of the AI problems mentioned in this blog. Where it is relatively easy to develop dataset as raw higher resolution, error-free data is available.
Gyrus provides AI Models as a Service. by working with customers to clean up, normalize and enrich their data for Data Science. Gyrus develops custom models based on the cleaned customer data and datasets/models.
Above all, Gyrus has worked with several customers in developing similar ML/AI algorithms for vision processing.
バックグラウンド
機械学習(ML)およびディープラーニング(DL)技術を備えた人工知能(AI)は、ビジネス、デバイスとそれらの使用法のあらゆる側面を変革することを約束します。 場合によっては、現在のプロセスを強化し、場合によっては、それらを置き換えることができます。
ビデオストリーミングデバイス(TVおよびSTBを含む)は、家庭内のAIアシスタントの1人になることを目指しています。 ただし、通常のストリーミングデバイスとビデオ処理には、AIの進歩によるTV変換に適したいくつかのタッチポイントがあります。
視覚的に強化されたモデルと使いやすさの観点から、エクスペリエンスの品質が向上すると予想されます。 これらの機能を備えたビデオストリーミングデバイスは、そうでないデバイスよりも確実に優れています。
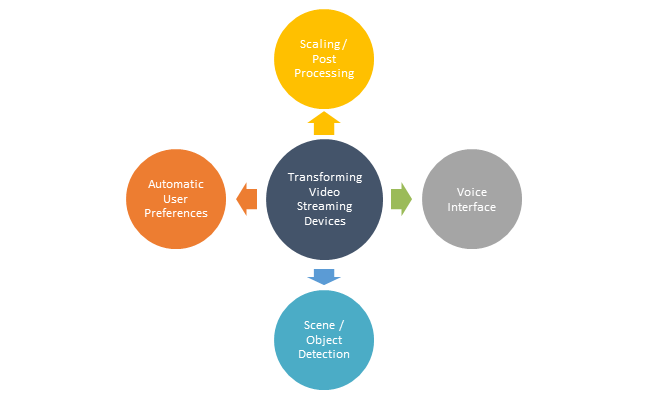
このブログでは、TVユーザーエクスペリエンスを向上させるためにビデオストリーミングデバイスで実行されるさまざまなML/AIモデルの使用について説明しています。
超分解能
超分解能は、アップスケーリングによって特定の低解像度から高解像度の品質を向上させる手法です。 一方、人々の嗜好は高品質で視覚的に魅力的な画像やビデオ品質に傾いています。
したがって、今日、超解像機能は多くのビデオストリーミング ディスプレイ デバイスに見られ、センサーのピクセル数の2〜4倍の解像度を生成します。
以下の写真の例からわかるように、ニューラルネットワークベースのスケーリングは、従来のバイリニアスケーリングよりもはるかに優れています。
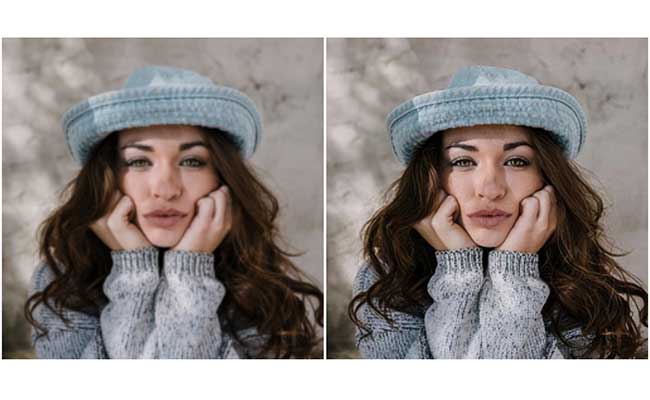
ニューラルネットワークモデルで拡大縮小された写真に見られるように、詳細は保持または強化されています。
スケーリングに使用するニューラルネットワークには、2つのタイプのモデルがあります–
•コンボリューション/デコンボリューション(Deconv)ベース
•GenerativeAdversarial Network(GAN)ベース
Deconvベースのモデルはより単純で一般化が優れていますが、GANベースのモデルはスケーリング関数に対して非常に優れたパフォーマンスを発揮するようです。
Deconvモデルには、ResNetなどの使い慣れたネットワークと同様の初期セクションがあり、画像のサイズは小さくなりますが、フィーチャの数が増えます。後半は、デコンボリューション操作を使用してフィーチャを使用して解像度を上げる拡張ネットワークです。 ネットワーク内の残りの接続は、より一般化するのに役立つようです。
Generative Adversarial Networks(GAN)は、2つのニューラルネットワーク(ジェネレーターとディスクリミネーター)が互いに決闘するシステムで構成されています。ターゲットサンプルのセットが与えられると、ジェネレーターは、ディスクリミネーターをだましてそれらが本物であると信じさせることができるサンプルを生成しようとします。 Discriminatorは、偽の(生成された)サンプルから実際の(ターゲット)サンプルを解決しようとします。
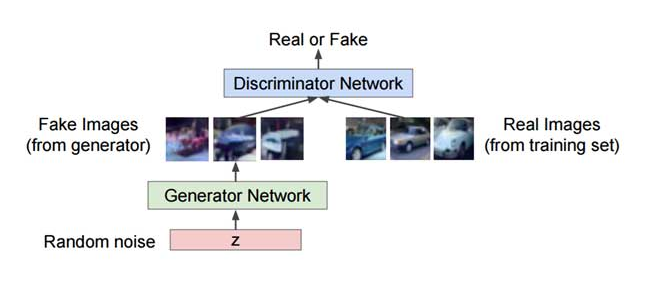
ニューラルネットワークによるInPainting
画像の一部が破損したり、以前の透かしで歪んだり、不明瞭になったりする場合がいくつかあります。 ニューラルネットワークは、画像の欠落している部分を推測することで、このような傷を非常にうまく修復できます。 超分解能と同様に、DeconvネットワークとGANを実現できます。
ただし、一般化が優れているため、Deconvネットワークの性能は向上しているようです。
単一ネットワークが、超分解能と修復(およびその他のノイズリダクション)の両方を一緒に行うようにトレーニングされています。
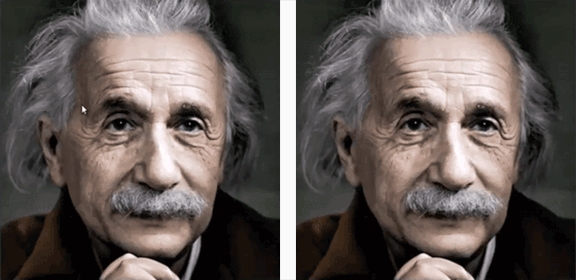
フレームレート変換
アニメーショングラフィックスの補間とアップスケーリングは、エッジがシャープであり、単純な補間では画像がぼやける傾向があるため、困難です。 これらの柔らかいエッジは、高解像度では視覚的に心地よいものではありません。 繰り返しになりますが、ニューラルネットワークは、フレームレート変換(およびアップサンプリング)において従来の方法よりも優れているようです。以下にリンクされている論文は、Variational Autoencoder、GAN、およびDeep Convolution Networksを使用してこの機能の実行方法を示しており、Deep ConvolutionNetworksは他の従来のアップサンプリングよりも優れたパフォーマンスを示しているようです。
テレビの統合ホームアシスタント
家庭用テレビのAI統合ビデオストリーミングデバイスは、家族が視聴中であることを認識し、各個人の好み、視聴習慣、特定の番組を追跡できます。
いくつかのアプリケーションがコンテンツを提供しているため、特定のプログラミングに合わせてアプリケーションを切り替えたり、メニューを閲覧したりするには、かなりの時間がかかります。
最新のNielsenレポートによると、平均的な視聴者は視聴コンテンツを選ぶのに7分かかります。視聴コンテンツを見つけるためにストリーミングサービスのメニューを閲覧していると報告しているのはわずか3分の1で、21%は決定できないため視聴をあきらめていると答えています。
バックグラウンドアプリケーションは、特定のユーザーを認識してこのタスクを実行できます。 テレビに接続されたデバイスを介したビデオ視聴は、主にそれらのデバイスの浸透と、古くて機敏性の低いセットアップの崩壊により、毎日8分増加しています。
現在、10世帯のうち7世帯がSVODサービスを利用しており、72%がビデオストリーミング対応のテレビデバイスを使用しています。 また、レポートは、何を見るべきかを選択することに関して、顧客は快適な環境にいないと主張しています。
テレビで実行されているバックグラウンドアプリケーションは、個人の好みや視聴習慣に従って特定のコンテンツを推奨するタスクを実行できます。
匿名化された人物の検出
上記のすべての機能は、ユーザープライバシーを侵害することなく、特定ユーザーのビデオ/顔などを記録することなく実行出来ます。
また、ソース側で着信ビデオストリーミングを匿名化し、ボール/スティックレベルで人物認識を実行するために、いくつかの新しいAI技術が利用可能です。 さらに、ニューラルネットワークは、プライバシーを侵害することなく、人の歩行に基づいてユーザーを識別します。
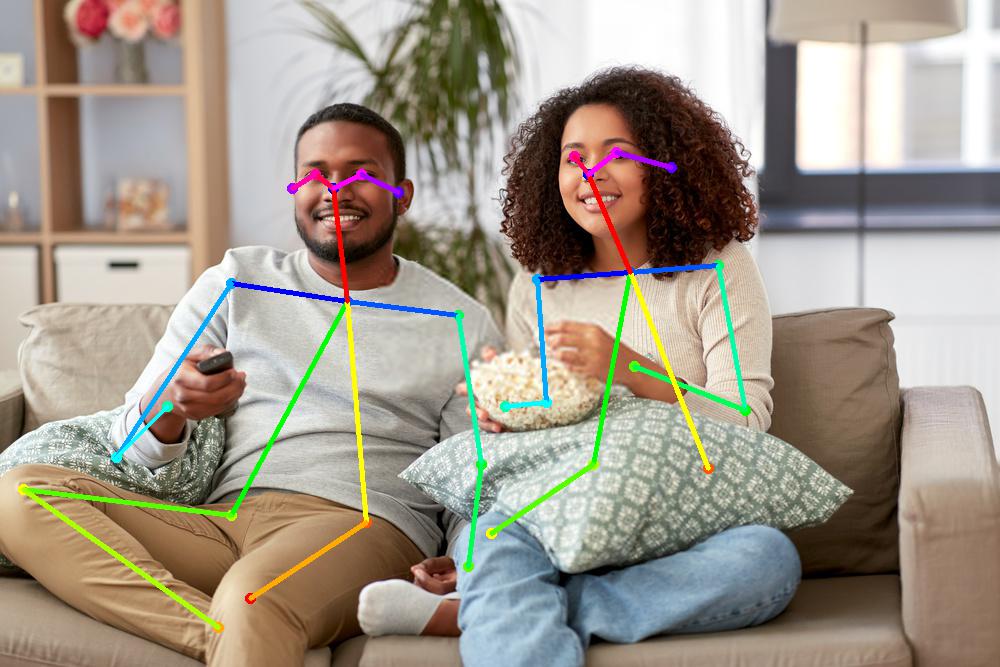
ノイズリダクション
後処理により、エンコーダのブロッキング、ブラー、リンギング、知覚量子化(PQ)によって引き起こされるノイズを除去し、PSNRを向上させることができます。
エンコーダーによって導入された障害は、非ブロックフィルターなどの特定のフィルターで除去されます。 ただし、ニューラルネットワークを使用すると、ノイズリダクションの性能を向上させることができ、すべてを1つのアルゴリズムで実行できます。
MEMC(Most Estimation and Motion Compensation)を実行するためのテレビの後処理オプション。また、熱心な批評家がいるので、ニューラルネットワークで強化することができます。

自動シーン検出
実行中の画像/シーンに関する情報を使用できるアプリケーションがいくつかあります。 特定のアクションを検出するためのビデオ分析を使用して、ビデオの特定のセクションを評価できます。 同様に、音声は言語評価(子供の校正用)について分析できます。
すべてのフレームは、特定のオブジェクト/場所の検出、キャラクターの顔認識、ヌードの自動分類のために分類できます。
このインサイトは、喫煙/飲酒などの規制警告の自動キャプションに使用できます。
ボイスコントロール
Amazon AlexaやGoogle HomeなどのHome AIアシスタントと非常によく似ており、自宅のビデオストリーミングデバイスは音声コマンドに応答することが期待されています。 今日、多くのリモコンはすでに音声起動しています。
高音出力デバイスを入力デバイスにするのは非常に複雑で、出力オーディオをキャンセルする必要があります。
さらに、外部スピーカーで音声を強調すると、周囲のノイズにさまざまな遅延が発生します。 今日、複雑なニューラルネットワークを使用して実装されたウェイクワード検出と自動音声認識(ASR)。 また、テレビの場合、コマンドを聞くには周囲のノイズを補正する必要があります。
結論
TV/STBに適切なニューラルネットワークアクセラレーションハードウェアを使用すると、上記の後処理のすべてまたは一部を実装して、現在の後処理と比較してユーザーエクスペリエンスを向上させることができます。
さらに、この処理により、半導体チップ設計者は、ニューラルネットワーク処理を実行するための統合ハードウェア要素の現在の高価なフィルターと後処理ハードウェアを廃止できます
AIアルゴリズム開発の問題の1つは、データセットの可用性と注釈です。
また、このブログで言及されているAIの問題も同様です。生(RAW)の高解像度としてデータセットを開発するのが比較的簡単な場合は、エラーのないデータを利用できます。
Gyrusは、AIモデルをサービスとして提供します。 お客様と協力して、データサイエンスのデータをクリーンアップ、正規化、強化します。 Gyrusは、クリーンアップされた顧客データとデータセット/モデルに基づいてカスタムモデルを開発します。
とりわけ、Gyrusは、視覚処理のための同様のML/AIアルゴリズムの開発において、複数の顧客と協力してきました。